Cluster Analysis and Validation in Unraveling Environmental Context of Non-Small Cell Lung Cancer Using Geometric Deep Learning of Satellite Imagery
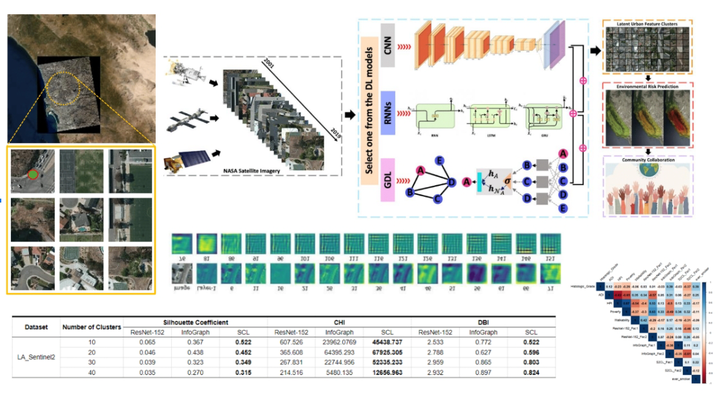
Supervisor: Jay Yang
The utilization of social determinants of health (SDOH) has enhanced the understanding of cancer disparities, yet the integration of urban-environmental characteristics at the neighborhood level remains partial. Satellite imagery, as a readily available data source, facilitates the observation and quantification of urban geographical contexts and their relation to environmental, socio-demographic, and health processes. Traditional deep learning (DL) methods, particularly Convolutional Neural Networks (CNNs), have been employed to analyze high-resolution images, focusing predominantly on local contextual information.
The advent of Geometric Deep Learning (GDL) presents novel methodologies for analyzing satellite data, specifically through superpixel graph representations, enabling a more nuanced understanding of urban environments. This paper introduces S2CL, a new superpixel simplicial contrastive learning model that leverages mutual information maximization between graph-level and simplicial complex-level representations. Conducting experiments in Los Angeles, USA, with lower resolution satellite imagery, we found that S2CL and GDL models surpass CNNs in revealing latent urban features, which complement SDOH variables in predicting cancer disparities.
Our findings suggest that GDL, particularly the S2CL model, provides a groundbreaking avenue for enhanced assessment of urban forms and their impact on health outcome disparities.